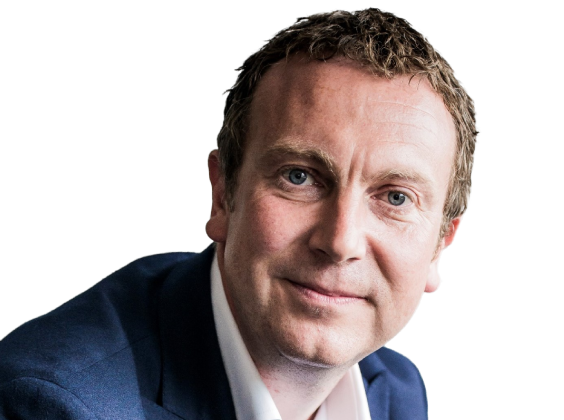
Written by
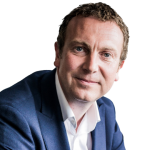
AI at RSNA – Signs of Maturity, But A Long Way to Go
AI was once again the hot topic at this year’s RSNA. With close to 60 AI start-ups in the Machine Learning Showcase and most of the incumbent modality and imaging IT vendors marketing AI to varying degrees, the technology was hard to ignore at this year’s meeting. Cutting through the inevitable hype, it was clear that vendors are making progress with bringing AI solutions into the daily workflows of radiologists and other imaging specialists. From our discussions with radiologists and from the general buzz on the show floor, it was also evident that the sentiment for AI has progressed from the initial fear and scepticism, to actively engaging with vendors to explore how AI can maximise productivity and lead to better clinical outcomes. While most now agree that AI is the future of radiology, it’s important to remember that we are still in the early stages of technology maturity, both in terms of technology readiness, deployment models and commercial strategies. The table below presents a maturity model for deep learning-based image analysis for medical imaging.
Product Evolution – Where do we go from Here?
Most of the AI solutions on show at RSNA were essentially detection and/or quantification tools for specific use-cases, e.g. lung nodule detection. Over time, these point solutions will evolve to have broader capabilities. For example, tools that provide automatic feature detection will also provide quantification and characterisation of abnormalities. There were a handful of solutions of this type on show at RSNA. For example, Koios presented its Decision Support (DS) solution for breast ultrasound that assesses the likelihood of malignancy based on the BI-RADS evaluation scale. Similarly, iCAD’s ProFound AI‚Ñ¢ for digital breast tomosynthesis (DBT) provides a Case Score on a percentage scale to represents how confident the algorithm is that a case is malignant. In 2019, we expect to see similar solutions for other pathologies, such as lung, liver, prostate and thyroid cancer.
Figure 1 – Deep Learning in Medical Image Analysis Maturity Model
In addition to disease characterisation, decision support features will increasingly be added to AI-based diagnostic tools, providing radiologists with access to automatically registered prior scans and case-relevant patient data from the EHR and other clinical systems, at the point-of-read. Early to market examples on show at RSNA included HealthMyne’s QIDS platform, which provides radiologists with a quantitative imaging dashboard including time-sequenced Epic EHR information, and Illumeo from Philips Healthcare. Illumeo is a primary reading tool that automatically adjusts hanging protocols and presents the radiologist with contextual patient information and relevant prior studies. Illumeo also suggests relevant tools and algorithms for the body area being examined. Another example of a decision support tool on show at RSNA is IBM Watson Imaging Patient Synopsis, a cloud-based solution that searches both structured and unstructured data in the EHR to find clinically relevant patient data, and then presents it in a single-view summary for the radiologist. Ultimately, today’s image analysis solutions will also provide differential diagnosis, but this is likely a few years away (Stage 4 of the maturity model).
As well as supporting a broader set of functionalities, today’s point solutions are also expected to evolve to body area and multi-organ solutions, that can detect, quantify and characterise a variety of abnormalities for entire body areas. While today’s point solutions are certainly of value in specific use-cases, particularly for high volume imaging such as cancer screening, they offer limited utility for general radiology as they solve a very small set of problems. To maximise the value of AI in medical image analysis, radiologists require solutions that can detect multiple abnormalities on multi-modality images. Moreover, body area solutions will likely be more cost-effective for care providers than purchasing multiple point solutions.
As point solutions evolve to body area solutions, this will unlock the full potential of AI in medical imaging by further accelerating read times and ensuring that incidental findings are less likely to be missed. For example, an AI-powered analysis of a chest CT ordered for a suspected lung condition may identify incidental cardiac conditions, such as coronary calcifications. The end goal here is an AI solution that can assess multiple anatomical structures from any modality, or even a whole-body scan.
There were several examples of body area AI solutions on show at RSNA. Siemens Healthineers chose RSNA to announce its AI-Rad Companion platform which takes a multi-organ approach to AI-based image analysis. The first application on the new platform will be AI-Rad Companion Chest CT, which identifies and measures organs and lesions on CT images of the thorax, and automatically generates a quantitative report. Another example is Aidoc’s body area solutions for acute conditions that detect abnormalities in CT studies and highlight the suspected abnormal cases in the radiology worklist. It currently has solutions for head and cervical spine, abdomen and chest applications. It has received CE Mark for the head and C-spine solution and FDA clearance for its head solution, although this is currently limited to acute intracranial haemorrhage (ICH) cases. An innovative approach to body area image analysis is Change Detector from A.I. Analysis, which detects changes in serial medical imaging studies. The company is preparing an FDA 510(k) application for a head MRI version of Change Detector and it intends to expand its use to support additional areas of the anatomy, for example breast cancer screening, and additional modalities.
Clinical Relevancy – Do We Need an App for That?
AI-based image analysis solutions for a wide range of radiology specialities, from neurology through to musculoskeletal, were on show at this year’s RSNA, with a particularly high concentration for lung and breast applications. However, health care budgets are under pressure globally and care providers will need to prioritise their AI investments by initially selecting a shortlist of vendors and algorithms to evaluate. In 2019, we expect to see the strongest market uptake for AI solutions that lead to improved clinical outcomes and deliver a return on investment for providers, either through productivity gains or by enabling more cost-effective diagnosis and treatment pathways. Examples include:
- Solutions for repetitive and high-volume tasks, such as cancer detection in screening programs.
- Triage solutions that prioritise urgent cases in the radiology worklist.
- Tools that provide automatic quantification to replace time consuming (and less repeatable) manual measurements.
- Solutions that facilitate the use of non-invasive imaging techniques, e.g. FFR-CT instead of coronary angiography.
In the short-term, AI tools that make radiologists more efficient and productive will be the low-hanging fruit, but in the longer run, the move to quantitative imaging and the use of imaging biomarkers to predict a patient’s response to treatment will become an increasingly attractive market. For example, quantitative data on a tumour’s characteristics such as its position in the organ and its heterogeneity, not just its size and shape, will enable more personalised, and potentially cost-effective, treatment planning. Moreover, by reviewing the treatment outcomes of patients who presented with similar disease characteristics, the most effective treatment plan can be selected for each patient. These solutions are likely to appeal to both care providers and payers alike.
Clinical Validation – Not all Algorithms Were Created Equal
There is little to differentiate the underlying deep learning technology behind most of the AI-based image analysis solutions on show at RSNA and it is typically the quality of the training data that dictates the performance of the machine learning model. When evaluating vendor solutions, care providers need to consider both the quantity, diversity (ethnicity, age, co-morbidities, etc.) and the quality of the ground truth data. A lack of images or inconsistent image annotation may compromise the performance of the algorithm during the training process. The approach to image annotation may also have a bearing on the performance of the algorithm, with some vendors using natural language processing (NLP) to mine radiology reports and others investing in more time consuming and expensive manual annotation of the pixel data. One of the most common reasons for a disconnect between the performance of algorithms during development versus deployment in a clinical setting is the quality of the validation dataset. Care providers are encouraged to ask the algorithm developer how the validation dataset was selected and if the data is from the same source/partner as the training data.
In addition, the results obtained from one patient cohort cannot always be generalised to others. For example, will an algorithm trained on data from Chinese patients work as expected on European or American patients, and vice-versa? Similarly, the training data must include images from a variety of modality vendors, scanner models and imaging protocols.
NVIDIA announced two new technologies at RSNA to assist algorithm developers with data curation and annotation – Transfer Learning Toolkit and AI Assisted Annotation SDK for Medical Imaging. The former enables developers to use NVIDIA’s pre-trained models with a training workflow to fine-tune and retrain models with their own datasets. The first public release includes a model for 3D MRI brain tumour segmentation. The AI Assisted Annotation SDK helps to automate the process of annotating images. It utilises NVIDIA’s Transfer Learning Toolkit to continuously learn by itself, so every new annotated image can be used as training data.
The market for AI-based medical image analysis tools is firmly in the early stages of development and the transition to it becoming a mainstream market is likely a few years away. With hype for AI at an all-time high, vendors need to undertake large-scale clinical validation studies to demonstrate the performance of their solutions in real-world clinical settings and to ascertain if the promise of workflow efficiency lives up to expectations, and by how much. This will boost radiologist trust and confidence in the technology and strengthen the business case for investing in AI solutions. Furthermore, without evidence of a clear ROI, the significant investment in software and the IT infrastructure required to support AI deployments will be hard to justify.
That said, there have been few such studies to date. At RSNA, RADLogics showcased a study from UCLA, published in Academic Radiology, that evaluated its Virtual Resident software integrated with PACS, configured to automatically incorporate detection and measurement data into a standardised radiology report (Nuance Powerscribe 360). The results indicate that radiologists’ time to evaluate a chest CT and create a final report was decreased by up to 44%. The study also found that the overall detection rate of the system was comparable to radiologists who did not use the software and nodule measurement was consistent between the radiologists as well as the software, with a variation of 1mm or less.
iCAD presented the results from a retrospective, fully-crossed, multi-reader, multi-case study that compared the performance of 24 radiologists reading 260 DBT cases both with and without its ProFound AI solution between two separate reading sessions. The findings showed that the concurrent use of ProFound AI improved cancer detection rates, decreased false positive rates and reduced reading time by 52.7% on average.
During RSNA, Aidoc announced a partnership with American College of Radiology (ACR) Data Science Institute, the University of Rochester Medicine and Nuance Healthcare to contribute to the recently launched ACR-DSI ASSESS-AI registry, which aims to provide post-market surveillance and continuous validation of AI performance and impact in real clinical settings.
Workflow Integration – It’s a Platform Play
Workflow integration remains one of the biggest challenges with bringing AI-based image analysis tools to market and there were a variety of integration flavours on show at RSNA. These ranged from dedicated AI platforms, both vendor-specific and vendor-neutral, that integrate with diagnostic viewers, workflow platforms and reporting tools, to direct integrations into the primary diagnostic viewing platform itself. While there are pros and cons of each, it’s becoming increasingly clear that for AI solutions to enter the mainstream market, they need to be easily accessible and introduce minimal disruption to existing workflows. Radiologists may be willing to accept one more application in the form of a dedicated, multi-vendor AI platform, but it is unrealistic to expect them to use multiple, vendor specific AI platforms. Each time a radiologist comes out of their primary platform to launch a vendor specific AI solution is time lost. This will be particularly hard to justify for AI platforms with a narrow offering of algorithms. Moreover, the huge integration effort associated with individually deploying vendor specific AI products will be prohibitive.
The big three medical imaging vendors – Siemens, Philips and GE – are essentially following the same AI deployment strategy – a dedicated AI platform coupled with an ecosystem of third-party applications. Fujifilm is pursuing the same strategy and Terarecon has the Northstar AI Explorer platform that integrates with its iNtuition AV platform and third-party PACS. Other examples of dedicated AI platforms on show at RSNA were Nuance’s AI Marketplace and Blackford Platform from Blackford Analysis. The other PACS and enterprise imaging vendors with AI solutions on show at RSNA typically integrated the algorithms directly into their diagnostic viewing platforms, although some are expected to launch dedicated AI platforms and/or AI marketplaces in the coming years.
Figure 2 – AI Platforms at RSNA
The mid-sized imaging IT vendors are likely to use a combination of direct integration of best of breed algorithms into their diagnostic platforms, supported by an AI marketplace for less commonly used algorithms that do not warrant a full integration. Smaller imaging IT vendors lack the resources for multiple direct integrations and will most likely partner with AI marketplaces.
In addition to software applications, there were a handful of examples of embedded AI solutions on show. Samsung showed a digital radiography system (GC85A) with embedded AI for bone suppression and lung nodule detection (FDA 510(k) pending) alongside its S-Detect‚Ñ¢ for Breast, an AI-based software which analyses breast lesions using ultrasound images, which is available on its RS80A ultrasound platform. GE Healthcare once again showed its Critical Care Suite (FDA 510(k) pending) embedded on the Optima XR240amx digital mobile x-ray system. Critical Care Suite automatically detects and prioritizes critical cases, the first example of which is an algorithm for pneumothorax. GE also showed SonoCNS Fetal Brain, a deep learning application that helps to align and display recommended views plus measurements of the foetal brain, on its Voluson E10 ultrasound system and Koios’ Decision Support (DS) solution for breast ultrasound embedded on its LOGIQ E10 premium ultrasound platform as a proof of concept.
It was somewhat surprising there were so few edge AI solutions on show at RSNA. Perhaps the modality vendors are keeping their powder dry for ECR? Either way, we expect edge AI to be a major trend in the coming years and across all modalities.
Figure 3 – AI Marketplaces at RSNA
Route to Market – AI Marketplaces an Attractive Proposition
As we mentioned in our RSNA 2017 show report (AI at RSNA – What a Difference a Year Makes), online AI marketplaces provide algorithm developers with workflow integration and a route to market. Despite the accelerated algorithm development times associated with deep learning compared with earlier feature engineering techniques, the cost to develop and commercialise algorithms is high. The commercialisation process is also lengthy due to the stringent regulatory process. For the incumbent imaging IT vendors, AI marketplaces are an effective way to bring a wide selection of AI-based image analysis tools to their customers without incurring high development costs. In the coming years, radiologists will have hundreds of algorithms at their disposal and it’s clear that no single vendor can do this on their own. Partnerships are essential. For care providers, AI marketplaces offer a “one-stop-shop” to a pre-vetted, wide range of AI-based tools that integrate directly into their diagnostic viewing or reporting platform.
Enterprise app marketplaces have proved successful outside of healthcare, for example, Salesforce’s AppExchange has several thousand enterprise apps and several million installs. Within the healthcare market the concept is also relatively proven, with the major EHR vendors like Epic, Cerner and Allscripts having opened their platforms to third parties and launched app stores. While the concept is yet to be proved in radiology, early customer wins from Nuance, Blackford Analysis and EnvoyAI are encouraging.
In addition to the marketplaces listed above, MDW (Medical Diagnostic Web) announced its medical imaging AI marketplace at RSNA. The MDW platform leverages blockchain technology and enables care providers to purchase algorithms and to monetise their imaging data by making it available to developers as anonymised, annotated datasets for algorithm training and verification. The platform is in beta release with general availability scheduled for Q1 2019.
NVIDIA announced that Ohio State University (OSU) Wexner Medical Center, an academic medical centre and university, is the first US partner to adopt its NVIDIA Clara platform to build an in-house AI marketplace to host native applications for clinical imaging. OSU demonstrated models for coronary artery disease and femur fracture on the NVIDIA booth. For care providers, the benefits of a home-grown marketplace are tighter integration into clinical workflows, a sandbox for internal algorithm development projects, ownership of feedback data for continuous learning and transfer learning, and tighter control of the third-party algorithms made available on the platform. We expect other academic hospitals with algorithm development activities to also develop their own marketplaces. Over time, provider owned marketplaces are likely to integrate with third-party marketplaces, such as those in the table above, to give radiologists access to the widest range of AI-based tools as possible.