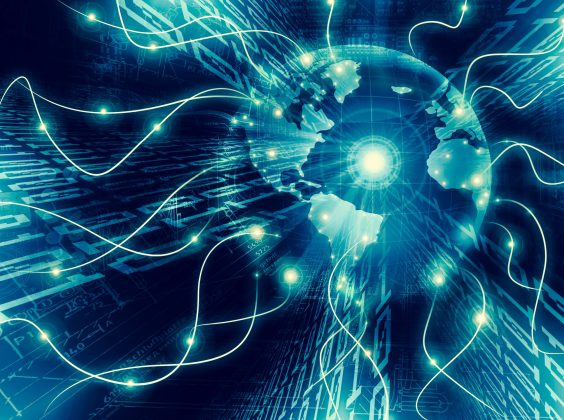
Written by

Introduction
In the previous Insight, the concept of generative AI (gen AI) was explained, and the gen AI value chain, highlighting its ecosystem’s focal point on major technology companies, was examined. Gen AI is a type of AI constructed upon a foundation model that undergoes extensive training on an expansive corpus of unlabelled data. This starkly contrasts conventional machine learning algorithms, which rely on labelled data and are tailored for specific task-oriented training.
The adoption of gen AI technology in medical imaging is primarily driven by its potential to address two major challenges plaguing the industry: staff shortages and clinician burnout. By integrating gen AI into medical imaging IT, there is the potential to streamline and expedite tasks, ultimately saving valuable time for clinicians. This not only enhances overall workflow efficiency but also serves as a crucial antidote to the pervasive issue of burnout among healthcare professionals. The automation and assistance provided by gen AI can significantly reduce the burden on clinicians, allowing them to focus on more nuanced aspects of patient care and decision-making, thereby fostering a healthier and more sustainable work environment within the medical imaging field. In February 2024, Signify Research published a report on vendor opportunities and strategies in medical imaging. This Insight offers a glimpse into the report’s findings, focusing on gen AI’s impact along the Medical Imaging value chain, as illustrated in the graphic below. I’ll start by exploring the infrastructure layer, then examine the model layer. Then, I’ll delve into the applications layer, discussing the potential impact of gen AI on modality vendors, imaging IT vendors, and reporting vendors.
Figure 1: Generative AI Value Chain
Infrastructure
The infrastructure layer of gen AI technology encompasses both hardware and cloud components. In terms of hardware, it involves the foundational technological infrastructure optimised for the training and inference of gen AI models. Concurrently, the cloud aspect involves on-demand access to computer system resources, encompassing data storage and computing power essential for the seamless operation of gen AI applications.
Big Tech
Big Tech has a pivotal role to play in providing the infrastructure segment of the technology stack. Providing this stack requires two assets; deep pockets, as the technology is expensive to run, and access to a lot of data, necessary for training the large models. Major tech players are engaged in fierce hardware competition. Amazon Web Services (AWS) introduced Inferentia and Trainium chips tailored for demanding AI tasks. Google used Tensor Processing Unit (TPU) for neural network machine learning since 2015. Microsoft Azure has not yet developed its own solution but has the world’s largest cloud investment in field-programmable gate arrays (FPGAs) using Intel solutions. OpenAI is reportedly exploring its AI chip development, entering the competitive landscape.
The healthcare industry widely adopts cloud technology, with Azure and AWS emerging as primary choices among providers. In contrast, Google holds a relatively smaller market share in this domain. Future changes in relationships with key health IT vendors, such as Epic’s collaboration with Google Cloud, may impact the choice of cloud service providers for advanced AI applications.
Model
Essential for generative AI, foundation models (FMs) come in various forms, including large language models (LLMs), multi-modal models (MMMs), and large vision models (LVM), and can be open-source or proprietary. Open-source models foster transparency and collaboration, allowing innovation without fees, while proprietary models, maintained by private companies, often feature cutting-edge technologies. Foundation Models, like GPT-4 (OpenAI), Amazon Titan (AWS), PaLM 2 (Google), and Claude 2 (Anthropic), are typically ‘domain-agnostic.’ However, a trend towards ‘domain-specific’ FMs, tailored for industries like healthcare, is emerging, with examples such as Google’s Med-PaLM-2 and Hippocratic AI. In healthcare, while AI adoption is increasing, domain-specific models are in the early stages. Currently, providers mainly use commercially available, proprietary, domain-agnostic models such as GPT-4, applied to a limited number of use cases.
In the medical imaging domain, the latest newcomer, HOPPR, aims to be the GPT of medical imaging by strategically aligning with AWS, leveraging its computing power and tool development kits. HOPPR is partnering with large healthcare systems to leverage their data and with vendors like Rad AI and RadNet to develop end-user applications. From the end-user perspective, HOPPR is prioritising collaborations with major industry players over individual radiologists or hospitals.
Another prominent player, Aidoc, which develops clinical AI solutions, is launching a major initiative with a $30 million investment to create a clinical AI foundation model, leveraging imaging data to enhance diagnostic precision and enable early detection of critical conditions, addressing key aspects of accelerated development, comprehensive coverage, and heightened accuracy.
Both foundation models are multi-modal, signalling a shift from language-centric to a comprehensive approach, reflecting the inherent multi-faceted nature of healthcare.
Application
The application layer, in the context of generative AI, encompasses a wide range of players involved in the medical imaging market, including modality vendors, imaging IT vendors, and reporting vendors. The integration of generative AI in this layer is expected to bring advances in efficiency, accuracy, and overall effectiveness, ultimately reshaping the landscape of medical imaging solutions.
Modalities
Modality vendors are preparing to deploy prototypes of gen AI to experiment with various cases and identify the most viable ones. The focus is on improving workflow efficiencies through internal use and short-term strategies such as workflow automation in areas such as consolidating patient history, layman translation, and enhancing efficiency at the point of image acquisition. In the pursuit of better patient health outcomes, vendors may explore using gen AI for precision medicine, leveraging enhanced interoperability facilitated by gen AI to integrate data from different departments.
Imaging IT
Imaging IT vendors are currently making short-term investments in business operations with a focus on enhancing efficiency. While avoiding grandiose claims, these efforts involve leveraging existing radiology and geographical footprints to introduce selected-gen AI services. Key considerations include identifying use cases for integration into the current product portfolio and determining the pricing strategy for these gen AI features. The strategic goal is to shift towards “cross-ology,” beginning with cardiology and expanding into other domains, mirroring the evolution in enterprise imaging. The decision-making process for partnerships, acquisitions, or in-house development hinges on assessing the return on investment and associated opportunity costs. Additional considerations for the imaging IT vendors are the regulatory hurdles associated with integrating gen AI into their product offerings. Although not crystal clear, as the FDA has not made any statements, regulators around the world will likely follow a conservative approach to regulating gen AI in the healthcare domain. Australia, for instance, has forbidden the integration of LLMs into any healthcare-related functions, including prior report summarisation.
Reporting
The excitement surrounding gen AI is particularly pronounced in the domain of reporting vendors, where the vision of achieving a fully automated radiology report is considered the pinnacle. The emphasis on this goal is indicative of the profound interest in automating segments of reporting, such as generating auto-impressions. Signify Research classifies automated reporting and prior report summarisation as use cases likely to occur, underscoring the significant anticipation in these areas.
While a prevailing argument suggests the potential commodification of the market, with Nuance currently dominating the scene, some may question the rationale behind investing in this technology. The concern is valid, as a saturated market and an unknown return on investment could potentially diminish the motivation of other vendors. However, it’s crucial to recognise the opportunity cost of the failure to adopt this technology, which may result in falling behind competitors. Despite the risk of commoditisation, embracing this technology becomes imperative for staying relevant and competitive in an evolving market landscape.
Conclusion
The landscape of gen AI in medical imaging presents a spectrum of possibilities, spanning from quickly attainable applications such as ambient listening, user assistance, to more complex endeavours like prior report summarisation and automated reporting. Notably, the latter endeavours pose heightened complexity due to higher barriers to entry, chiefly regulatory constraints. As Big Tech giants engage in fierce hardware competition and companies like HOPPR and Aidoc strategically align with major players, innovation is set. The shift from LLMs to MMMs hints at a dynamic future in generative AI development. Vendors addressing the application layer, from modality vendors to imaging IT and reporting vendors, anticipate advancements in efficiency and accuracy. Yet, amidst this promise, the question looms: What challenges and opportunities will arise as generative AI takes centre stage in medical imaging? Vendors aiming for success must meticulously plan their focus, balancing short-term objectives with medium-term goals. If not already in consideration, vendors should contemplate the integration of gen AI solutions into their product roadmaps to safeguard against falling behind. Disregarding this strategic alignment poses the risk of rapid obsolescence in the continually advancing field of medical imaging.
This Insight serves as an introduction to Signify Research’s Generative AI Market Intelligence Service, laying the groundwork for a comprehensive analysis. Going beyond informative reports, this service provides access to a robust product database. This database facilitates a clear comparison of announced generative AI applications, offering a streamlined understanding of prominent use cases and competitive vendors. Tailored to empower decision-makers, this resource delivers valuable insights to guide strategic decision-making.
Related Research
Generative AI Market Intelligence Service 2024
This Market Intelligence Service delivers data, insights, and thorough analysis of the worldwide market potential for vendors leveraging Generative AI in HealthTech. The Service encompasses Medical/Clinical IT, EMR & Digital Health, Pharma & Life Sciences, and Big Tech vendors, exploring their opportunities and strategies in the realm of generative AI.
About The Author
Jon joined Signify Research in 2023 as a Market Analyst in the Digital Health team. He holds a BSc in Pharmacology having graduated from the University of Bath in 2023.
About the Digital Health Team
Signify Research’s Digital Health team provides market intelligence and detailed insights on numerous digital health markets. Our areas of coverage include electronic medical records, telehealth & virtual care, remote patient monitoring, high-acuity clinical information systems, patient engagement IT, health information exchanges and integrated care & value-based care IT. Our reports provide a data-centric and global outlook of each market with granular country-level insights. Our research process blends primary data collected from in-depth interviews with healthcare professionals and technology vendors, to provide a balanced and objective view of the market.
About Signify Research
Signify Research provides healthtech market intelligence powered by data that you can trust. We blend insights collected from in-depth interviews with technology vendors and healthcare professionals with sales data reported to us by leading vendors to provide a complete and balanced view of the market trends. Our coverage areas are Medical Imaging, Clinical Care, Digital Health, Diagnostic and Lifesciences and Healthcare IT.
Clients worldwide rely on direct access to our expert Analysts for their opinions on the latest market trends and developments. Our market analysis reports and subscriptions provide data-driven insights which business leaders use to guide strategic decisions. We also offer custom research services for clients who need information that can’t be obtained from our off-the-shelf research products or who require market intelligence tailored to their specific needs.
More Information
To find out more:
E: enquiries@signifyresearch.net
T: +44 (0) 1234 986111
www.signifyresearch.net