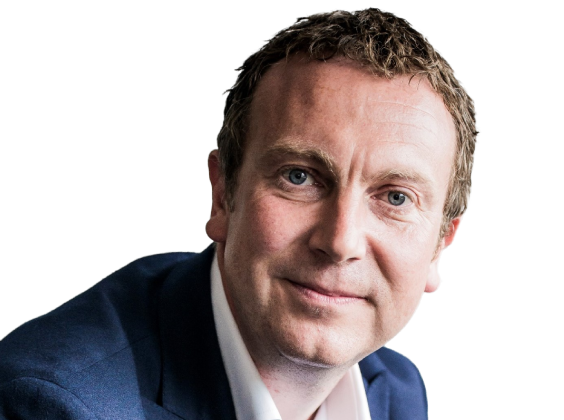
Written by
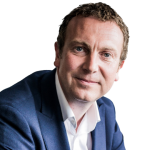
Machine learning was undoubtedly one of the hottest topics in radiology last year, with a steady stream of academic research papers highlighting how machine learning, particularly deep learning, can outperform traditional algorithms or manual processes in certain use-cases. Investment in machine learning start-ups also continued, with several companies attracting early stage funding. To date, more than $100m has been invested in start-ups that are developing AI solutions for radiology. Furthermore, commercial activity gained pace, with at least 20 companies exhibiting AI-based products at the RSNA conference towards the end of the year, although most were prototypes and only a handful had regulatory clearance. 2017 should see commercial activity ramp-up, FDA approvals permitting.
Whilst the enthusiasm for machine learning is certainly justified, it inevitably raises expectations, potentially to unrealistic levels. To counter this, machine learning companies must clearly articulate the value proposition of their solutions and demonstrate a clear return on investment (ROI) to healthcare providers. With healthcare budgets under pressure globally, vendors should demonstrate both improved clinical outcomes and a tangible ROI to stand the best chance of success. As a bare minimum, vendors must prove that any quality improvements from using their cognitive tools do not negatively impact clinician productivity.
Using cognitive tools for repetitive and time consuming tasks enables radiologists to focus on value-added tasks or to perform extra reads. Machine learning can be used to extract relevant information from an EHR or lab report to automatically populate the radiologist’s report. The same cognitive tool can present the radiologist with treatment outcomes from similar cases, to aid with diagnosis and treatment planning. In this example, the combination of increased radiologist productivity and improved clinical outcomes makes for a compelling argument for healthcare providers to invest in machine learning.
A commercially available example of how automated tools can lead to productivity gains is 4D Flow from Arterys. 4D Flow uses cloud-based image processing technology to provide visualization and quantification of blood flow on cardiac MRI studies. With 4D Flow, cardiac MRI exam times can be significantly reduced from typically 60 to 90 minutes to around 10 minutes, which increases the efficiency and throughput of the hospital’s MRI service. Additionally, automated segmentation eliminates the need for radiologists to calculate measurements between areas of the heart. Cardiologists will need to switch from using echocardiograms, but the benefit of more accurate flow data that can be tracked over time should be a motivator. Arterys also recently received FDA 510(k) clearance and CE Mark approval for its Cardio DL‚Ñ¢ product that provides automated, editable ventricle segmentations based on conventional cardiac MRI images, eliminating the need for manual segmentation. Cardio DL‚Ñ¢ is also cloud-based and leverages deep learning technology.
Another example is iCAD’s PowerLook¬Æ Tomo Detection, a Computer Aided Detection (CADe) system for breast tomosynthesis that’s built on deep learning technology. Each image in a tomosynthesis data set is analysed to detect potential areas of interest and the system blends those areas onto a synthetic 2D image so that they are visible on a single image of the breast. Based on initial trials, the company claims that the additional reading time associated with breast tomosynthesis over 2D mammography is reduced by an average of 29.2%, with no change in radiologist performance.
In the above examples, the ROI from using cognitive analytical tools is largely derived from radiologist (and technician) productivity gains. However, bigger opportunities are to be found higher-up the healthcare value chain. For example, cognitive tools can review existing scans to identify incidental findings that have not been followed-up and that could represent missed billing opportunities for the healthcare provider. Moreover, predictive analytics can identify at-risk patients to enable early intervention and to avoid costly readmissions, both leading to a reduction in treatment costs.
Whether machine learning is positioned as a productivity play, a cost-saving play or a revenue-generating play, one thing is clear – vendors must prove the ROI. Unsubstantiated claims of how machine learning can outperform or replace radiologists may attract the news headlines but do little to support the efficacy of the technology. Vendors must adopt a more responsible, fact-based approach to marketing their solutions if machine learning in radiology is to avoid the “trough of disillusionment”.
New Market Report from Signify Research Publishing Soon
This and other issues will be explored in full in Signify Research’s upcoming market report ‘Machine Learning in Radiology – World Market Report‘, publishing in January 2017. For further details please contact simon.harris@signifyresearch.net