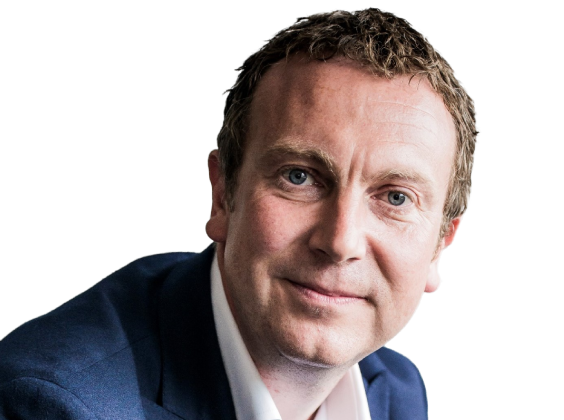
Written by
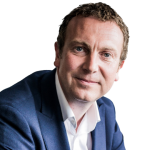
For companies developing machine learning solutions for radiology, strategic collaborations and partnerships with healthcare providers are king. Not only do they provide vendors with academic and clinical domain expertise, but they also give access to annotated imaging data to train and validate machine learning algorithms – one of the biggest challenges for most algorithm developers. By working closely with providers, vendors can identify the greatest pain-points for physicians, be it workflow-related or part of the clinical decision-making process, and hence the areas where machine learning can add the greatest value.
To that end, leading medical imaging companies are forging strategic partnerships with health providers to collaborate on machine learning projects, as shown in our graphical analysis below.
GE Healthcare Partners with Partners
One of the most recent was last week’s announcement from GE Healthcare and Partners HealthCare that they have entered a 10-year collaboration to develop artificial intelligence and deep learning solutions. Partners is a Boston-based, not-for-profit health care system that was founded in 1994 by Massachusetts General Hospital and Brigham and Women’s Hospital. Key points from the announcement are as follows:
The collaboration aims to implement AI into every aspect of a patient’s journey through the healthcare system – from admittance through discharge – and spans multiple medical specialties, including radiology, pathology, genomics and population health.
- The initial focus of the relationship will be on the development of applications aimed at improving clinician productivity and patient outcomes in diagnostic imaging.
- Early applications will address cases like determining the prognostic impact of stroke, identifying fractures in the emergency room, tracking how tumours grow or shrink after the administration of novel therapies, and indicating the likelihood of cancer on ultrasound scans.
- GE and Partners will co-develop an open platform to enable them and third party developers to rapidly prototype, validate and share applications with hospitals and clinics around the world.
Strategic Partnerships for Machine Learning Radiology
GE Healthcare, IBM Watson Health, Siemens Healthineers and Philips Healthcare
Note: blue linear lines indicate vendor partners and green leader lines indicate clinical partners
Source: Company annoucements
GE Healthcare has existing partnerships with Boston Children’s Hospital, to develop solutions that interpret paediatric brain images faster and more accurately, and with UC San Francisco’s Center for Digital Health Innovation. The partnership with UCSF aims to develop a library of deep learning algorithms, with an initial focus on algorithms that expedite differential diagnosis in acute situations such as trauma.
GE Healthcare also has partnerships with several image analysis vendors, including Arterys and imbio, whose solutions are made available on GE Health Cloud. The solutions developed by GE and its clinical partners will also be available on GE Health Cloud, creating a large library of image analysis applications.
Watson Health Medical Imaging Collaborative Now at 24 Members
IBM Watson Health is also implementing a partnership strategy and in June 2016 it announced the Watson Health Medical Imaging Collaborative. The initiative launched with 16 members, including health systems, academic medical centres, ambulatory radiology providers and imaging technology companies, and has since expanded to 24 organisations. The first application from the collaborative is IBM Watson Imaging Clinical Review, which helps hospitals to identify patients that may have aortic stenosis. Sentara Heart Hospital contributed data from 3,000 heart echo-cardiology studies, 60% of which were used, to train Watson in the development of IBM Watson Imaging Clinical Review. The collaborative is also developing solutions for diseases of the eye, brain and other heart-related conditions such as myocardial infarctions, valve disorders, cardiomyopathy and deep vein thrombosis.
IBM Watson Health is also courting partnerships with image analysis vendors for Watson Platform for Health (formerly Watson Health Core), its healthcare data platform-as-a-service offering, powered by Watson Health Cloud, which features a mix of IBM Watson Health and third-party applications. One of the first third party radiology products is an application from MedyMatch that detects intracranial bleeds from CT scans.
Siemens Launches Digital Ecosystem
Siemens Healthineers announced its new digital healthcare platform, Digital Ecosystem, at HIMSS 2017 and has partnered with several third-party software developers, including Arterys, HeartFlow and SyntheticMR. In January this year, Siemens and Biogen announced an agreement to jointly develop new MRI tools for quantifying key markers of multiple sclerosis disease activity and progression, including new T2 lesions and atrophy.
Siemens has announced relatively few machine learning partnerships with health providers. At RSNA 2016 it presented a prototype developed with Essen University Hospital to improve the diagnostic accuracy of a non-expert radiologist in differentiating between usual interstitial pneumonia (UIP) and non-UIP on thoracic CT. Siemens is also a partner in the ‚ÄòData Intelligence for Clinical Solutions’ project, funded by the Federal Ministry for Economic Affairs and Energy (BMWi), which aims to develop artificial intelligence solutions that provide physicians with differential diagnoses based on available patient data. The clinical partners in the project are Charit√© hospital in Berlin and Erlangen University Hospital.
Philips Focuses on its In-house AI Capability
Philips Healthcare has several long-term, strategic partnerships with health providers, typically based on a managed services business model. Examples include Westchester Medical Center Health (US), Heart Hospital Tampere (Finland) and Karolinska Hospital (Sweden). However, these partnerships typically do not have specific projects on machine learning. One exception is a deal signed in March this year with Phoenix Children’s Hospital for a 15-year agreement relating to imaging, patient monitoring and clinical informatics, which will explore the use of machine learning in paediatric care.
Similarly, Philips has relatively few partnerships with image analysis software vendors and to date has not pursued the ‚Äòopen imaging ecosystem’ approach of GE (Health Cloud), IBM (Health Core) and Siemens (Digital Ecosystem). Instead, Philips appears to be more focused on developing its in-house artificial intelligence capability. At RSNA 2016 it introduced Illumeo, which uses data and contextual awareness to help optimise radiologist workflow. Illumeo adapts the user interface by offering tool sets and measurements driven by the understanding of the clinical context. The software provides the radiologist with the most relevant case-related information from various sources in a single view and generates dynamic reports that can include 3D images or image quantification data.
Not the Only Game in Town
This article has focused on the partnership activities of a handful of the leading medical imaging companies. There is also considerable partnership activity from mid-tier imaging companies looking to add machine learning to their repertoire and the growing number of start-up companies in this field. Moreover, open platforms are available from other vendors, e.g. NTT DATA Unified Clinical Archive, and it is likely than new ones will come on stream in the coming years. Specialist image analysis platforms are also available, such as those from medimsight and McCoy Medical, although these are perhaps better suited to clinical research than mainstream clinical practice.
More Partnerships Still Needed
One of the most commonly cited shortcomings of machine learning in radiology is the limited number of commercially available products. Most vendors offer only a handful of solutions, which are typically focused on a specific application area, e.g. breast, lung or cardiology, which limits the utility of machine learning for most radiologists. Generalist radiologists require a comprehensive “analytical tool kit” with a broad portfolio of algorithms. It’s a daunting task for a single company to create such a library of algorithms, comprising tens and potentially hundreds of analytical tools. Instead, the radiology industry must continue to collaborate for radiologists, and ultimately patients, to fully benefit from the rapid developments in the field of machine learning.