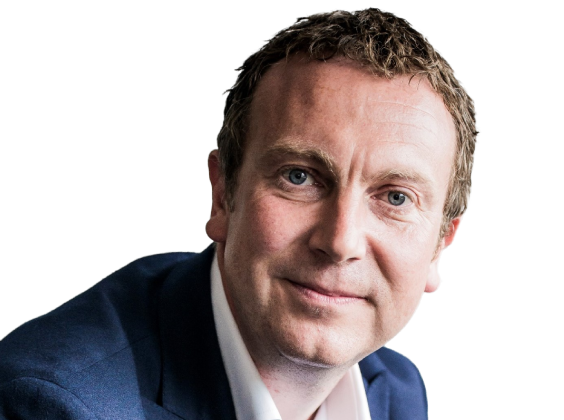
Written by
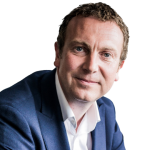
Advances in technology and the increase in population continue to apply pressure to radiologists across the world. Although the quality of the images in increasing, so is the quantity; there were ~22.5 million x-ray images taken by the UK NHS in 2016. This becomes even more of an issue when you look at the plateau in the number of radiologists, leading to an increase in time for x-ray analysis; 230,000 patients waited longer than one month for x-ray results within the UK NHS in 2016. The same trend is evident in many other countries around the world.
But general radiography x-ray images only produce a fraction of the volume of data when compared to CT or MRI scans. CT and MRI scans make up such a large portion of the images taken because of both the quantity and the quality of the images. It is within these imaging modalities where disease detection and diagnosis are the most challenging, and human error is the greatest concern. Therefore, it is understandable why most of the companies who are developing machine learning algorithms for medical imaging are focused on MRI and CT, rather than x-ray images. But are there use cases where machine learning can be utilised in general radiographic image analysis, to fulfil a specific need within the healthcare sector?
X-rays are used to diagnose a wide range of conditions, with the most common procedures being extremities, abdomen/pelvis and chest. With chest x-ray procedures accounting for around half of all x-rays taken, this represents the highest volume application, and hence the best potential for a return on investment for algorithm developers. Listed below are some examples:
Enlitic’s deep learning software focuses on image analysis, and covers a wide range of use cases from patient triage to processing insurance and pharmacy data. In China earlier this year, Enlitic partnered with Paiyipai to implement its deep learning technology on the millions of x-rays accumulated from yearly check-ups carried out in health centres across China.
Research carried out in La Fe Polytechnics and University Hospital, and presented at ECR this year, used deep learning in a computer-aided detection (CAD) system to help radiologists perform automated screening of chest x-rays. This increases the efficiency of the process and prioritises abnormal images for analysis. In testing, the machine produces 83% sensitivity and 80% specificity for abnormal cases.
Delft DI (part of Canon) uses machine learning for computer aided detection of TB in developing countries, where TB is prevalent but there is an acute shortage of trained radiologists. The CAD4TB software reads digital chest radiographs looking for abnormalities consistent with TB, and Delft DI claims the software is now more accurate than a human reader (although not as accurate as a specialist physician).
Riverain Technologies uses machine learning and imaging processing algorithms for a slightly different outcome, but has also been showed to have a positive impact on x-ray anomaly identification. The Riverain ‚ÄòClearRead Bone Suppress’ software suppresses the ribs and clavicles in chest X-ray images, which often obscure visibility, making it more difficult to detect abnormalities or nodules in the lung that could indicate diseases such as cancer. The software gives healthcare professionals an unobstructed view of the lungs to help increase lung disease detection rates.
EXINI Diagnostics has identified an alternative use case to chest x-ray, utilising artificial neural networks to detected tumour growth within the human skeleton from x-ray images. The spread of prostate cancer into the skeletal bones can be quantified to identify the tumour burden on the patient, but manual image reading and interpretation is subjective. The EXINI software is used as an imaging biomarker to quantifiably identify ‚Äòhotspots’ from bone density scans where the healthy bone has been overtaken by the tumour. Although it is unclear how EXINI plans to commercialise the software following the acquisition by Progenics Pharmaceuticals in October 2015.
IBM Watson is being used to facilitate a more holistic approach to diagnosis. By the end of 2017, IBM plans to commercialise its ‚Äòcognitive data summarization tool’, which analyses a patient’s individual healthcare background, including results from other imaging studies, for a more informed interpretation of the medical images.
There are also a number of machine learning companies still at the research and development stage of utilising deep learning to detect and aid in diagnosis from radiographic images:
- Predible Health is developing a deep learning application to detect musculo-skeletal disorders from x-ray images.
- Lunit use a deep learning algorithm to analyse and interpret x-ray images. The software is involved in disease diagnosis in both chest radiography and mammography.
There is definitely a need for machine learning to aid the detection of anomalies within CT and MRI scans, and there is software capable of doing just that. But is there a need to roll out machine learning across all general radiography procedures? Probably not. Machine learning is capable of being implemented in a wide variety of x-ray medical imaging analysis; from identification of broken wrists to better facilitating the radiologists view of the lungs through bone suppression. But the more important question is not if the software can be utilised, but if the healthcare providers can see a need for the software in the real-world environment, and are prepared to pay for it.
In the longer term, the implementation of machine learning in CT and MRI has highlighted an important point for other modalities: machine learning is best utilised to handle the meta-data of a patient as they are treated for a disease. The software has shown it could be invaluable for tracking, processing and prioritising the huge amounts of information generated. From detection of an abnormality, to tracking a lesion, monitoring risk factors and bringing a personalised treatment plan to the patient; the best use of machine learning in general radiography looks to be associated with the diagnosis and monitoring of diseases, particularly within oncology.