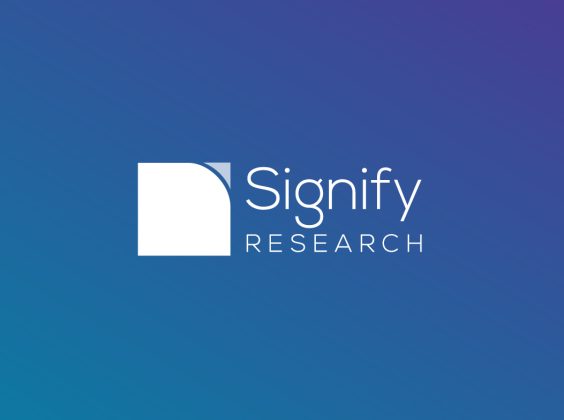
Download the Full Analysis here.
The market for AI in drug development and clinical trials is maturing and entering a new phase; total funding in the industry has reached $5.2B, but investments have slowed down in 2019 and only 4 new companies were formed. Any new start-ups will have to prove themselves able to compete against well-funded first-generation vendors, and existing vendors are under pressure to demonstrate results. Signify Research has analysed the investment landscape and categorised the industry into Information Synthesis, Drug Design and Clinical Trials. To download the full investment analysis in pdf format, click here.
Which Applications are AI Used For?
Nine out of ten new drug candidates never make it through clinical trial and regulatory approval, so the pharmaceutical industry is very open to new ways that can make drug development more efficient. The focus of this analysis has been on applications that are directly involved in optimising drug development by analysing clinically relevant data to guide the discovery of new potential targets, applications that are directly involved in the creation and optimisation of the molecular structure of potential drugs, and applications that help organise, optimise, run and recruit patients for clinical trials.
Information Synthesis – To aggregate and synthesise information is fundamental to AI applications being used in both drug design and clinical trials. Platforms are typically delivering broad data analysis and predictive models based on machine learning and deep learning algorithms. This category captures vendors using AI to analyse clinical data and real-world evidence across different applications, which can then be used in clinical trials or drug design. Any integrated information synthesis functionalities in drug design or clinical trial applications are instead included in each of those categories.
Drug Design – Compared to the other two product segments included in this investment analysis, start-ups developing AI for drug design are leading in terms of number of companies and total amount of funding so far, and several new drugs are currently going through clinical trials. AI is generally being applied to identify patterns hidden in large volumes of data or calculating the effect of small molecular iterations to optimise and predict efficacy and specificity. Within this field, the applications are often interrelated and include using AI to analyse clinical, scientific, patient and genomic data to get a better understanding of disease mechanisms, and using this to generate either novel drug candidates or to repurpose existing drugs for new diseases and therapeutic uses. Once potential targets have been identified AI is also being used to accelerate the drug design process by performing in-silico experimentation and conformational analysis on drug molecules to gain insights into the behaviour and physical properties of the molecule prior to in-vitro and in-vivo testing.
Clinical Trials – Clinical trials is an area with great potential for optimisation as only 12% of drug development programmes ended in success in a 2000-2019 study on clinical trial failure rates. Inability to demonstrate efficacy or safety, flawed study design, participant drop-outs or unsuccessful recruitment all contributed to the low success rate of clinical trials. Vendors active in this field are therefore focusing use of AI around three main areas: 1) Natural language processing to enable analysis and decision making from structured and unstructured data from medical records, relevant guidelines, real-world data and other sources that could potentially enhance the quality and efficiency of clinical trials. 2) Clinical trial design and optimisation pulling in information from comparable studies, clinical data and regulatory information to optimise eligibility criteria, cost, length and retention. 3) Clinical trial recruitment using natural language processing to analyse doctor’s notes and clinical data such as pathology reports and patient history in medical records to determine the pool of potential subjects, or help clinicians find relevant clinical trials and treatments for their patients by analysing electronic medical records and genomic data for improved clinical trial matching.
Within these three product segments, 32% of funding has gone to companies focusing on Information Synthesis, 54% to companies focusing on Drug Design and 14% to companies with a primary focus on Clinical Trials.
AI in Drug Development vs AI in Medical Imaging
AI in drug development and clinical trials has seen tremendous initial investment. Compared to AI in medical imaging the total investment has been more than four-fold, even though the number of funded start-ups is equivalent (101 funded start-ups in medical imaging against 106 funded start-ups in drug development and clinical trials). With 231 deals in medical imaging and 303 deals in drug development, the average deal size in drug development is 3.5 times bigger than in medical imaging.
AI in medical imaging and AI in drug development are two very different markets with each their characteristics. While medical imaging is very well suited for the use of machine learning-based pattern recognition, adoption within healthcare providers is a notoriously slow process due to a lack of trust in AI amongst clinical staff, a value proposition which is difficult to prove, complex data integration due to siloed and proprietary imaging platforms, not to mention the challenging and uncertain regulatory approval process.
Despite requiring more complex solutions, AI in drug development has seen much more interest from the investment community. As big pharma is struggling with profitability due to high R&D costs, low success rate for new drugs, difficulty in enrolling patients for clinical trials and pressure on companies from governments to cut prices on pharmaceuticals, the industry is searching for new ways to reduce the cost of drug development and regain profitability. Not working in a hospital care setting, takes away some of the regulatory challenges and the complex go-to-market strategies. So, once the technologies have proven themselves ready for market with successful drug candidates and clinical trials, investors are expecting high returns through partnerships with the pharma industry.
AI in Drug Development – More Than Empty Promises?
While the number of companies founded in year 2017 peaked at 28, only 4 new companies were formed in year 2019. In addition, total funding decreased by 23% from 2018 to 2019. Ambitions and expectations have been sky-high for AI use in this sector, but some investors may now start to wonder if they had been too bullish and if the technology is ever going to deliver what has been promised. Even if AI would be able to assist with such a complex task as understanding the cause of disease, it would need access to vast amounts of data that is often not accessible or simply does not exist yet. So, as the market matures past the initial hype, investors, as well as potential new pharma partners, are waiting to see more evidence and proof of concept to demonstrate the functionality and value of these AI solutions.
However, there is already a lot happening in the industry with several AI designed drugs in the pipelines. Partnerships with big pharma vendors will be key to success for start-ups and will support turning proof of concept into potential milestone payments for new drug candidates, sometimes exceeding a billion dollars as seen with the InSitro-Gilead partnership, Exscientia-Celgene partnership and the Atomwise-Hansoh Pharma partnership. And, in addition to revenue, as pharmaceutical companies often are sitting on years of digitised data from research and clinical trials, the pharma partnerships will provide access to a larger pool of data to train the algorithms and discover new valuable connections in disease and treatment mechanisms that wouldn’t have been possible otherwise with traditional methods.
For the full analysis including geography, clinical, funding type and partnership segmentation, click here.