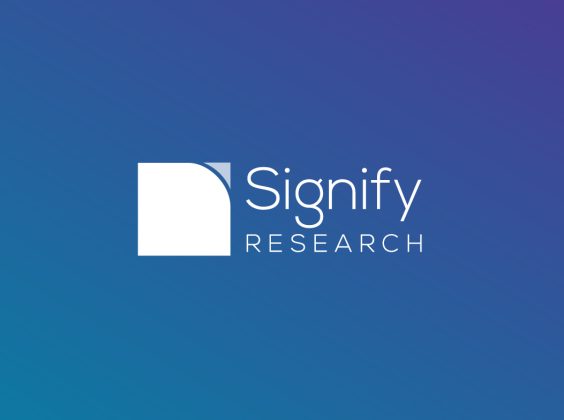
The application of machine learning in radiology is evolving at a rapid pace and whilst fully automated diagnostic systems are still several years away, there is a growing number of machine learning tools available now that are helping radiologists to improve their efficiency and to make better diagnoses. This Analyst Insight from Signify Research describes the current applications of machine learning in radiology and points to how the technology will increasingly be applied in four distinct areas, as shown the diagram below.
Computer Aided Diagnosis
Computer-aided detection (CADe) systems are intended to identify a variety of cancers such as breast cancer, prostate cancer, and lung lesions. They are most commonly used to detect microcalcifications and masses on screening mammograms. Despite concerns regarding the benefits of CADe and the high rate of false positives and false negatives, the market has grown steadily over the last two decades, most notably in the US where more than 90% of mammograms are interpreted using CADe. This has largely been driven by the availability of reimbursement for the use of CADe in breast screening. It is far less commonly used for detecting other cancers, where reimbursement for using CADe is currently not available.
Most CADe systems are rules-based and programmed to identify specific features. However, machine learning techniques, particularly deep learning using convolutional neural networks, are increasingly being applied. Deep learning has the potential to improve the accuracy of CADe, particularly for soft-tissue analysis. Moreover, as deep learning systems can be trained to identify features using large datasets, the algorithm development times are massively reduced compared with the traditional approach of ‘manually-crafting’ algorithms.
The benefits for radiologists are likely to be enhanced product performance (i.e. fewer false alerts), a faster pace of product innovation and a wider choice of products, particularly for the detection of lung, colon and prostate cancers, where there are currently only a handful of products on the market. For breast cancer detection, there will likely be a wider selection of CADe solutions for use with different modalities, e.g. breast MRI and breast ultrasound, in addition to digital mammography. In breast tomosysnthesis, deep learning can be applied to reduce the additional reading time associated with 3D images compared with 2D mammograms. For example, in March this year iCAD released PowerLook Tomo Detection which is built on deep learning technology. The system extracts areas of interest from the 3D planes and blends them onto a single 2D image. iCAD claims that its new system can reduce the interpretation time for breast tomosynthesis by an average of 29.2%.
Lastly, the increasing use of machine learning will enable CADe to gradually evolve from purely detection systems, to more advanced decision support and computer-aided diagnosis, as described in the following sections.
Quantitative Analysis Tools
Quantitative analysis tools are essentially workflow tools that provide radiologists with automatic detection and measurements of imaging features (biomarkers) to assist with diagnosis, such as lung density, breast density, analysis of coronary and peripheral vessels, etc. Much like with CADe, machine learning is increasingly being applied and the benefits to radiologists are much the same – improved accuracy, enhanced functionality and an increasing choice of products due to the faster algorithm development times. Most vendors currently offer a small number of quantification tools for very specific tasks, but the faster time to market associated with machine learning algorithms will enable vendors to offer an expanded ‚Äòtoolkit’, with solutions for multiple applications across multiple modalities.
Zebra Medical Vision, an Israeli start-up applying machine learning to medical imaging, has announced several algorithms for its Imaging Analytics platform. Its algorithm for lung applications analyses Chest CT scans to detect emphysematous regions in the lungs, and quantifies the volume of emphysema in comparison to the overall lung volume. Another example is 4D Flow from Arterys, which uses a cloud-based image processing technology to provide visualization and quantification of blood flow on cardiac MRI studies. 4D Flow utilizes machine learning analytics for automatic identification and segmentation and Arterys is validating the use of deep learning algorithms.
Decision Support Tools
Decision support tools provide detection and quantification, alongside supporting information extracted from an EHR, pathology reports and other patient records, to assist with diagnosis. Other features may include:
- Registration algorithms, to pinpoint areas of interest in one study and have those areas linked to the same points in previous scans,
- Automatic population of radiology reports with quantitative data,
- Predictive analytics to identify high risk patients and enable early treatment
- Treatment planning to determine the best course of treatment for an individual, by reviewing the outcomes of previous treatment pathways for patients with similar conditions.
Decision support tools do not provide automated diagnosis (see next section) and instead are intended to help radiologists improve their efficiency, while also improving accuracy and consistency. Early detection of high risk patients and improved treatment planning can also lead to cost savings for health providers and improved quality of care.
Today there are relatively few imaging decision support tools on the market, but this is likely to be one of the main applications for machine learning in radiology in the coming years. At this year’s RSNA meeting, HealthMyne will release its Quantitative Imaging Decision Support (QIDS) platform which combines imaging data with electronic medical record, radiation therapy and other clinical information to provide clinical decision support in the primary read. Another example is AlphaPoint‚Ñ¢ from RADLogics, which was launched in early 2016. AlphaPoint is a cloud-based platform that incorporates machine learning algorithmic tools for automatic analysis of images and merges the results with the patient’s medical record information in a preliminary report.
IBM Watson Health is currently developing a radiology assistant product, code-named Qibo, that reviews imaging studies and patient records to produce a summary of the most important information.
Computer Aided Diagnosis
Computer aided diagnosis (CADx) systems are intended to provide information beyond detection and quantification by also providing interpretation of the scan, for example by providing a probability score for the presence of cancer. These systems are heavily regulated by the FDA and as far as Signify Research is aware, there are no commercially available CADx systems for clinical use.
Several companies are developing fully automated diagnostic systems and probably the most ambitious is IBM Watson Health, whose project is code-named Avicenna, after an 11th century philosopher who wrote an influential medical encyclopedia. The key difference between Avicenna and existing decision support tools, as described in the previous section, is that Avicenna has a “reasoning” system that makes use of multiple data sources, such as scans, patient records and data from similar cases, to suggest diagnoses and possible treatment paths. IBM has shown previews of Avicenna, but has not indicated when it will be commercialized. Other companies developing CADx solutions include Aidence, Enlitic and CureMetrix, to name a few.
To summarize, we expect that 2017 will be a pivotal year for machine learning in medical imaging as the FDA is expected to approve the first tranche of detection and quantification tools based on deep learning. Although the clinical benefits of these systems are somewhat unproven, the early results from validation trials are encouraging. We also expect that several companies will release decision support tools which will help with market education as to the benefits of these systems. In the US, the trend to value-based care will be a major driver for their uptake. Looking beyond 2017, the rapid advancements in artificial intelligence technology, primarily driven by technology giants such as Apple, Google, Facebook and IBM, suggest that the importance of machine learning in radiology will only increase over time. That said, there are still legal and ethical considerations that need to be addressed, notwithstanding proving the efficacy of the technology, before computer-aided diagnosis becomes mainstream.
New Market Report from Signify Research Publishing Soon
This and other issues will be explored in full in Signify Research’s upcoming market report ‘Diagnostic Analytics in Radiology – World Market Report‘, publishing in January 2017.