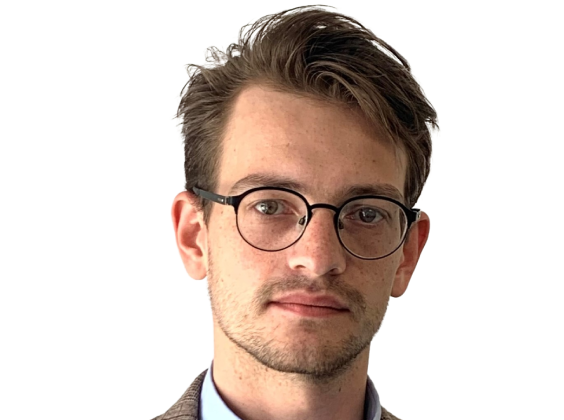
Written by
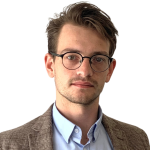
Cranfield, UK, 12th February 2024 – In the first Insight, we defined what generative AI (gen AI) is and discussed the gen AI value chain and how the ecosystem is centred around big tech. To recap, gen AI is an AI built on a foundation model trained on a large corpus of unlabelled data. In contrast, traditional machine learning algorithms use labelled data and are trained to perform a specific task.
You can download a copy of this third Gen AI insight or simply continue reading.
Figure 1: Generative AI Value Chain
This series of Insights focuses on the applications of general AI solutions. This is a precursor to a more in-depth analysis of Signify Research’s Generative AI Market Intelligence Service and this is our third instalment of the series. As you may remember from the first Insight, the care network concept was introduced with three stakeholders: the patient, provider and payer. The interconnection between them serves as the application areas for gen AI, summarised in the diagram below. From the care network, we can identify three end-user groups with similar use cases. These end users are patients, clinicians, and institutions.
Figure 2: The Care Network
The use case clusters around these end-user groups are presented in the graphic below.
Figure 3: End User Groups and Use Case Clusters
Following on from the previous Insight that looked into the Patient view and related gen AI applications, this insight will look at the possible applications from the Clinician’s perspective, while the next Insight will delve into the perspective of the Institution.
Helping the Clinician
In the healthcare landscape, the patient journey is intricately mapped across three essential stages: pre-care, in-care, and post-care. The pre-care phase encapsulates the critical activities conducted before a patient’s formal admission or before a patient-provider encounter, encompassing tasks such as scheduling, patient intake procedures, and obtaining necessary authorisations. During this stage, healthcare providers diligently prepare for the upcoming interaction, ensuring a streamlined and efficient process upon the patient’s arrival. Conversely, the in-care stage involves providing medical services and covering diagnosis and treatment efforts. Within this phase, healthcare professionals’ collaborative efforts come to fruition as they address immediate health concerns. The post-care stage traditionally denotes the period following treatment, marked by rehabilitation, monitoring, and ongoing support. However, in a dynamic healthcare environment, some individuals experience a unique shift, where post-care becomes a form of pre-care, signifying a transition to alternative care pathways.
The Clinician represents the use cases around providing care to the patient by a dedicated member of the healthcare provider team, whether it is a radiologist, a doctor, or a nurse. Broadly speaking, there are two categories of use case clusters: Clinical Workflow Support and Clinical Decision Support. As the name suggests, Clinical Workflow Support refers to the use of technology, tools, and processes to streamline and enhance the efficiency of clinical workflows to assist healthcare providers in managing and optimising the sequence of tasks and activities involved in patient care, whereas Clinical Decision Support relates to applications that provide timely information and diagnostics at the point of care to inform patient care decisions. Clinical Decision Support tools can relate to detection and diagnostics, where they would be categorised as Software as a Medical Device (SaMD) by the FDA and be regulated by MDR in the EU. Population Health Management (PHM) Clinical Decision Support is categorised as part of the Institution’s end-user group and hence will be covered in more detail in the following Insight.
Figure 4: End User Group – The Clinician
For this reason, we propose a framework outlined below that encompasses the distinction between CDS that are SaMD and the ones which are not, referred to as “Golden Mean”, because although they are primarily used for clinical decision support, they also improve the workflow process and improve efficiency. A good example is Wolters Kluwer’s integration of generative AI in UpToDate, which provides clinical decision support and improves efficiency and saves clinicians’ time at the point of care. The table below also shows common use cases around these use case clusters segmented by the care stages. The Clinical Decision Support, implicitly be definition can only happen at the point of care, explaining the absence of example use cases.
Figure 4: Use Cases – The Clinician
Clinical Workflow Support
Clinical Workflow Support is the most promising area of value development in the short and medium term. This area stands out due to its lack of inherent regulatory hurdles, making it ripe for automation of repetitive tasks that contribute to clinician burnout.
Pre-Care Stage Advancements
In the pre-care stage, generative AI proves instrumental in automating homogenous and repetitive tasks. Use cases such as scheduling, patient intake, and prior authorisation benefit significantly from streamlined processes. Athenahealth, for instance, has successfully integrated a generative AI solution for prior authorisation, enhancing the accuracy of insurance claims forms and improving the likelihood of prompt insurance pay outs for patients.
In-Care Innovations
Ambient Listening has emerged as a game-changer in the in-care stage. Many healthcare providers are transitioning from human scribes to machine-automated solutions. In collaboration with Microsoft, Nuance is leveraging OpenAI’s ChatGPT to enable faster and smoother notetaking at the point of care, something Epic has already taken advantage of. Integrating generative AI in coding translation will follow suit, translating patient conversations into applicable CPT codes and streamlining clinicians’ workflows.
Auto Impression summarises radiology reports, providing concise impressions of key findings; a notable example is Rad AI’s product that debuted in 2021. Future developments in this area are expected to focus on improved latency and personalised responses tailored to individual clinician writing styles. In the field of radiology, the pinnacle of success for Artificial Intelligence (AI) and Machine Learning (ML) lies in the automation of report generation based on diagnostic findings. Building on the concept of auto impressions, this represents a significant leap forward in AI system development. The automation of this tedious process for radiologists holds the promise of allowing them to review a larger volume of reports daily. This efficiency can have positive financial implications at the individual radiologist level, particularly given the prevalent per-read reimbursement model. Conducting more reads not only addresses staff shortages but also serves as an incentive for more professionals, such as radiologists and technicians, to join and contribute to the field.
Post-Care Optimisations
At the post-care stage, discharge summaries become a crucial tool for saving clinicians time and effort, particularly for patients with prolonged in-care periods. Generative AI aids in creating concise summaries, eliminating the need for discharging clinicians unfamiliar with the case to sift through vast amounts of data.
Additionally, the implementation of generative AI in follow-up procedures, especially in fields like radiology, proves to be a valuable use case. Machine learning algorithms identify patients at higher risk, enabling automated personalised outreach for additional checks and ultimately improving patient outcomes.
Golden Mean
From the unregulated realm of Clinical Decision Support, the integration of generative AI has been particularly driven by the imperative need for document summarisation. Notably, Wolters Kluwer has seamlessly incorporated this functionality into their UpToDate clinical evidence repository, empowering clinicians with more comprehensive information for informed decision-making at the point of care. Looking ahead, Electronic Health Record (EHR) vendors are actively pursuing the direct integration of this summarisation capability into EHR systems; Oracle, for example, has announced that this will be a feature of its next-generation EHR. This integration empowers healthcare providers to efficiently navigate extensive patient data, emphasising the most pertinent information.
Clinical Decision Support
Within the regulated framework of Clinical Decision Support, Signify Research does not anticipate a significant contribution from generative AI in the short- to medium-term. The absence of standardised regulations in this arena is a driving factor behind this cautious outlook. The ongoing efforts of the FDA to establish effective regulatory measures for generative AI suggest that applications in this space will likely remain at the research and development (R&D) level.
A noteworthy example of progress in this realm is ELIXIR, a model developed by Google. ELIXIR combines images with extensive language models, offering clinicians the ability to interrogate image data. Looking ahead, we can anticipate the evolution of such demonstrations, where an X-ray image, for instance, could trigger an automatic report generation, serving a diagnostic purpose and falling under the regulation as a Software as a Medical Device (SaMD). However, given the gradual pace of regulatory developments, the realisation of these use cases may take between 5 to 10 years.
It is essential to acknowledge the possibility that this level of automation might face resistance from radiologists and other clinicians, potentially limiting the adoption and return on investment for technology vendors. Despite this, as a proof of concept, we remain optimistic about the exciting prospects for the development of these applications in the years to come.
Conclusion
Generative AI stands to revolutionise the role of clinicians by automating routine tasks, offering workflow support, and enhancing decision-making processes. This has profound implications for improving patient care and outcomes. The table below offers a snapshot of recent developments aligned with the proposed framework. As healthcare embraces generative AI, its transformative impact on clinical workflows becomes increasingly apparent, spanning from pre-care to post-care stages. These technologies demonstrate versatility in enhancing efficiency, reducing burnout, and ultimately elevating patient care outcomes. Ongoing collaboration between healthcare providers and AI developers holds the promise of continuous advancements in applying generative AI within the healthcare sector.
Figure 6: Vendors Offering Solutions for the Clinician
This Insight serves as an introduction to Signify Research’s Generative AI Market Intelligence Service, laying the groundwork for a comprehensive analysis. Going beyond informative reports, this service provides access to a robust product database. This database facilitates a clear comparison of announced generative AI applications, offering a streamlined understanding of prominent use cases and competitive vendors. Tailored to empower decision-makers, this resource delivers valuable insights to guide strategic decision-making.
Related Research
Generative AI Market Intelligence Service 2024
This Market Intelligence Service delivers data, insights, and thorough analysis of the worldwide market potential for vendors leveraging Generative AI in healthtech. The Service encompasses Medical/Clinical IT, EMR & Digital Health, Pharma & Life Sciences, and Big Tech vendors, exploring their opportunities and strategies in the realm of generative AI.
About the Author
Vlad joined Signify Research in 2023 as Senior Market Analyst in the Digital Health team. He brings several years of experience in the consulting industry, having undertaken strategy, planning, and due diligence assignments for governments, operators, and service providers. Vlad holds an MSc degree with distinction in Business with Consulting from the University of Warwick.
About the Digital Health Team
Signify Research’s Digital Health team provides market intelligence and detailed insights on numerous digital health markets. Our areas of coverage include electronic medical records, telehealth & virtual care, remote patient monitoring, high-acuity clinical information systems, patient engagement IT, health information exchanges and integrated care & value-based care IT. Our reports provide a data-centric and global outlook of each market with granular country-level insights. Our research process blends primary data collected from in-depth interviews with healthcare professionals and technology vendors, to provide a balanced and objective view of the market.
About the Signify Research
Signify Research provides healthtech market intelligence powered by data that you can trust. We blend insights collected from in-depth interviews with technology vendors and healthcare professionals with sales data reported to us by leading vendors to provide a complete and balanced view of the market trends. Our coverage areas are Medical Imaging, Clinical Care, Digital Health, Diagnostic and Lifesciences and Healthcare IT.
Clients worldwide rely on direct access to our expert Analysts for their opinions on the latest market trends and developments. Our market analysis reports and subscriptions provide data-driven insights which business leaders use to guide strategic decisions. We also offer custom research services for clients who need information that can’t be obtained from our off-the-shelf research products or who require market intelligence tailored to their specific needs.
To Find out More:
E: enquiries@signifyresearch.net
T: +44 (0) 1234 986111
www.signifyresearch.net