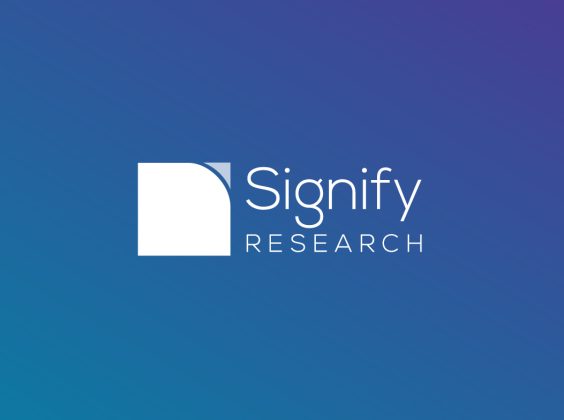
Written by
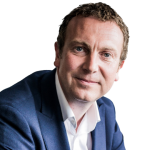
AI was undoubtedly the belle of the ball at RSNA 2017, and rightly so. As the meeting drew to a close, a report by the Care Quality Commission (CQC) in the UK revealed that a NHS hospital had a backlog of 23,000 chest x-rays acquired over the previous 12 months that hadn’t been formally reviewed. The hospital blamed a shortage of radiologists. Whilst AI is not the answer to all of radiology’s problems, it has the potential to improve both the speed and the accuracy of radiologists. If, hypothetically, this NHS hospital had medical imaging AI systems in place, perhaps some of the patients who were wrongly diagnosed would have received better care.
Whilst there is certainly a strong case for the increased use of AI in medical imaging, there are several challenges that first need to be overcome, including the availability of curated training data, the black box nature of deep learning, radiologist acceptance, workflow integration and an effective route to market, not to mention the opaque and expensive regulatory requirements. It was evident at this year’s RSNA that vendors had made good progress with bringing AI solutions to market. This article reviews the progress made, with product examples.
It’s All About Workflow
For algorithm developers, one of the major challenges is how to bring their solutions into the clinical workflow, without impacting radiologist productivity. Radiologists need results fast and readily accessible. Some may also want to review and edit the results before they are captured in a PACS or enterprise imaging solution.
Several of the medical imaging AI start-ups have developed cloud-based platforms for their applications, which are positioned as PACS agnostic for integration into the existing workflow. However, many seem to be downplaying the challenges and nuances associated with integrating AI platforms with different vendors’ PACS systems, without compromising the performance and robustness of the PACS. Platform developers need to openly address these issues and develop solutions to support PACS integration and the deployment of algorithmic tools.
Several companies showed AI platforms at this year’s RSNA. Arterys announced MICA, its cloud-based AI platform for medical image analysis. MICA currently supports three applications: the FDA-cleared Cardio AIMR and new clearance-pending applications to support oncology workflow for lung and liver: Arterys Liver AIMR, CT and Arterys Lung AICT. The announcement signals that Arterys is looking to replicate its success in cardiac MRI in other pathologies and with other modalities, with a view to offering a suite of analytical tools on the MICA platform.
HealthMyne’s Quantitative Imaging Decision Support (QIDS)¬Æ platform aims to impact clinical productivity and patient management decisions by providing multidisciplinary care teams (radiology and oncology today, with other major specialties going forward) with quantitative imaging data and supporting patient information in an intuitive way within their clinical workflows. QIDS supports quantification of lesions and lymph nodes in multiple body sites, including lung, brain, liver and kidney, and across multiple modalities, such as CT, MRI and PET, and leverages EMR, PACS, Radiation Therapy, and other clinical systems in a patient-centric clinical dashboard. Once the radiologist has identified a lesion, the Rapid Precise Metrics (RPM)‚Ñ¢ functionality displays suggested contours. After the radiologist has verified the contours on multiple slices, the quantitative metrics are calculated and flow to the radiology report in a tabular format, so there is no need to dictate numbers. These metrics include the long and short measurements along with other evidence-based metrics such as volume, density, mass, %GGO, and textural data that are tracked longitudinally for consistency. Over 400 quantitative metrics are available for current and future analysis. QIDS also supports incidental findings management, preparation for tumour board, clinical trial protocols, and ACR reporting such as Lung-RADS.
As radiology transitions to a value-based care model and precision medicine gains more traction, AI platforms such as QIDS and MICA will enable radiologists to demonstrate value by providing the referring physicians with objective, repeatable and data-rich reports. Other companies with AI platforms on show at RSNA 2017 were Lunit, RadLogics and Aidoc, to name a few.
Terarecon demonstrated Northstar, a dedicated viewer for radiologists to review the results from AI algorithms, which is due to be launched in March. By giving radiologists an opportunity to reject or edit the results from AI tools before they are committed to PACS, Northstar may help to alleviate radiologist concerns over the accuracy of AI and to mitigate potential legal implications. Terarecon also announced a distribution partnership with EnvoyAI to sell and market the EnvoyAI platform (see next section).
It was clear from our discussions with the medical imaging AI companies at RSNA that good progress has been made with workflow integration, at least on paper. However, it remains to be seen if AI systems will perform as expected in ‚Äòreal-world’ PACS deployments.
New App Stores Open for Business
Another of the key AI-related themes at this year’s RSNA was the launch of several online AI marketplaces. These marketplaces address two of the key challenges for algorithm developers – workflow integration and route to market. For health providers, the benefits of AI marketplaces are a single contract and a single integration, with a preveted selection of tools from specialist developers. Moreover, AI marketplaces provide radiologists with a wider selection of algorithms than any one developer can offer, at least in the short-term. An important point considering the many different diagnoses (more than 2,000) that radiologists make, compared with the limited number of algorithms available from most developers.
EnvoyAI, formerly McCoy Medical Technologies, announced the launch of the EnvoyAI Exchange, a medical imaging AI platform that launched with 14 signed distribution deals with partner companies, collectively contributing 35 algorithms to the platform. Three of the algorithms have FDA clearance. McCoy was acquired in June by TeraRecon, and has been spun-out to continue operations as an independent entity. EnvoyAI Exchange is integrated with Terarecon’s product suite, giving both EnvoyAI and its partners access to Terarecon’s installed base of more than 2,000 sites. Some of the companies signed-up to the EnvoyAI platform are 4Quant, Aidoc, icometrix, Imbio, Infervision, Lunit, Quibim, Qure.ai and VUNO.
Nuance also unveiled an online AI marketplace, called the Nuance AI Marketplace for Diagnostic Imaging. Through the Nuance AI Marketplace, technology partners can deploy their algorithms into the PowerShare Network, a cloud-based platform for accessing and sharing imaging data across organisations. The algorithms can then be selected and accessed through the PowerScribe reporting platform in a radiologist’s daily workflow. Nuance launched the marketplace in conjunction with numerous partners, including Aidence, the American College of Radiology, Lunit, NVIDIA, Ohio State University, RadLogics and Zebra Medical Vision. Nuance claims that 20,000 radiologists already use its PowerScribe radiology reporting software, which represents a sizeable market for its marketplace partners to tap into.
Blackford Analysis launched its Blackford Platform at RSNA. The platform hosts products from multiple partners, including A.I. Analysis, Aidoc, icometrix and Rcadia. The company recently announced that its Blackford Platform will be rolled out in eRAD PACS systems at more than 300 RadNet imaging centres across the US.
The major medical imaging vendors have been slow to roll-out clinical AI applications, which has left the door open for the dedicated medical imaging AI marketplaces to establish a first to market advantage. In the short term we expect these marketplaces will gain some traction, as health providers increasingly experiment and evaluate AI solutions. However, with the major vendors now ramping-up their AI activities and launching their own open ecosystems, as described in the next section, these online marketplaces may find it increasingly hard to differentiate and compete in what is currently a very small market.
Major Imaging Vendors are Ramping up on AI, but Cautiously
It wasn’t just startup companies who were flying the AI flag at this year’s RSNA – the major imaging vendors were also heavily promoting their AI activities. In most parts of the world, the imaging modality and imaging IT markets are well established with limited growth opportunities. Hence vendors need to innovate, both to defend their existing installed base, protecting recurring service and maintenance revenues, and to tap into new revenue streams. AI is enabling the major vendors to maintain their reputation for innovation and to introduce new intelligent solutions to retain their customers and encourage upgrades.
Whilst the medical imaging AI startups are mainly focussed on developing clinical applications for detection, visualisation, quantification and decision support, the major vendors are taking a different approach with an initial focus on applying analytics and AI to workflow and reporting tools. Most have R&D projects underway to also develop AI clinical applications, but recognise this is a longer-term play with regulatory challenges. By focusing on workflow and reporting tools, which typically do not require regulatory clearance, they are able to capitalise on the buzz around AI and position themselves as innovators in this field.
GE Healthcare demonstrated several analytics and machine learning enabled features at RSNA, positioning itself as supporting its customers from business intelligence, through to operational efficiency and clinical support. One of the machine learning features was Imaging Related Clinical Context (IRCC), which was developed in partnership with University of Pittsburgh Medical Center (UPMC). IRCC provides radiologists with relevant patient information in context, including EMR data, in their existing workflow. IRCC, which is expected to be available in 2018, learns from semantic feedback how to best select and present relevant clinical data to radiologists. GE’s Smart Reading Protocols (SRP) uses deep learning to ensure each radiologist’s protocols are always automatically hung in the same way. SRP learns reading tendencies to automate image setup and to launch advanced visualisation and other applications. With similar products already available from other vendors, e.g. Illumio from Philips, this was more a case of GE playing catch-up than innovating.
In addition to these workflow tools, GE also demonstrated two clinical applications; an algorithm being developed with UCSF that detects pneumothorax on X-ray images and a stroke detection algorithm being developed with Partners Healthcare. Both applications prioritise cases in the radiologist worklist based on abnormal findings and are planned for commercially launch in Q4 2018.
GE also reinforced its commitment to a ‚Äòbuild and partner’ AI strategy by announcing a partnership with MedyMatch, whereby MedyMatch will integrate its intracranial haemorrhage detection software into GE’s CT scanners. Shortly before RSNA, echocardiography image analysis developer DiA Imaging Analysis announced a multiyear, nonexclusive licensing and professional services agreement with GE Healthcare. GE’s other AI partners include Arterys, Imbio and Pie Medical.
Philips made several new product announcements at RSNA and plans to renew 60% of its Diagnostic & Treatment portfolio in 2017 and 2018. Whilst AI was certainly a marketing theme on the Philips booth, there were few tangible examples. Illumeo, which began shipping in mid-2017, remains the flagship AI product. Illumio provides a single view of the most relevant case-related information from various sources and adapts its user interface based on the preferances of the radiologist by offering tools and measurements to meet the clinical context of the images being interpreted. At RSNA 2017, Philips announced a collaboration with Nuance, whereby Illumeo will be integrated with Nuance’s PowerScribe 360.
Although Philips has indicated that it plans to offer applications from third party developers, it has yet to announce any partnerships. Instead the focus seems to be on in-house development and acquisition. In July, Philips announced the acquisition of TomTec Imaging Systems (click here for our insight on this deal) and shortly before RSNA it announced the acquisition of Analytical Informatics, a provider of workflow tools and applications. The Analytical Informatics acquisition will strengthen Philips’ PerformanceBridge portfolio of operational performance solutions. We expect that Philips will start to add AI partners in 2018 and develop an imaging ecosystem on its HealthSuite platform.
Siemens Healthineers also demonstrated several analytics and machine learning solutions, both for workflow, operational efficiency and clinical applications. On the clinical side, it demonstrated a new AI tool for its syngo.via advanced visualisation platform that automatically segments the lung, the heart and the aorta. It also showed Digital Ecosystem, a cloud-based platform based on its teamplay solution. Digital Ecosystem features teamplay’s analytical tools, such as fleet utilisation statistics, alongside applications from third party vendors, including SyntheticMR, Arterys, mint medical and Pie Medical Imaging. Users of syngo.via can access Digital Ecosystem using OpenApps.
Other AI announcements from the imaging modality and imaging IT vendors included:
- Sectra announced that its enterprise imaging platform now supports vendor-neutral access to machine learning solutions. The company plans to offer its own machine learning applications as well as third party applications.
- Agfa HealthCare announced that its plans to integrate third party applications alongside algorithms developed in-house in its Enterprise Imaging solution. The company announced new partnerships with RadLogics and Mindshare Medical. Agfa is also a member of the IBM Watson Health medical imaging collaborative.
- Fujifilm previewed some home-grown AI solutions that are under development, such as predictive tools for neuro imaging, and hinted that a medical imaging AI ecosystem with third party applications is on the way.
- NTT Data Services introduced an enterprise imaging analytics platform and announced a partnership with MD.ai. The platform also features tools from Imbio and Zebra Medical Vision.
Despite the Progress, Challenges Remain
Access to radiologist annotated data remains a major challenge for many algorithm developers, who must be prepared to invest significant time and money in data curation. Companies with innovative strategies for obtaining data will have a big advantage. Regulatory approval remains the other major hurdle in the commercialisation of deep learning solutions. The US FDA has approved very few deep learning solutions and a lack of guidelines is slowing the approvals process. A few more have obtained CE Mark, including Aidoc which announced it had received CE Mark for its deep learning solution for head and neck imaging at RSNA. Zebra Medical Vision also has CE approval for its Deep Learning Analytics Engine.
Several of the medical imaging AI startups who exhibited at RSNA had already submitted applications to the regulators or were in active discussions. With over 30 medical imaging AI start-ups exhibiting at this year’s RSNA (and even more that were absent), as well as AI solutions from many of the major medical imaging vendors, the availability of deep learning solutions for medical imaging is set to notably increase in 2018 regulators permitting.