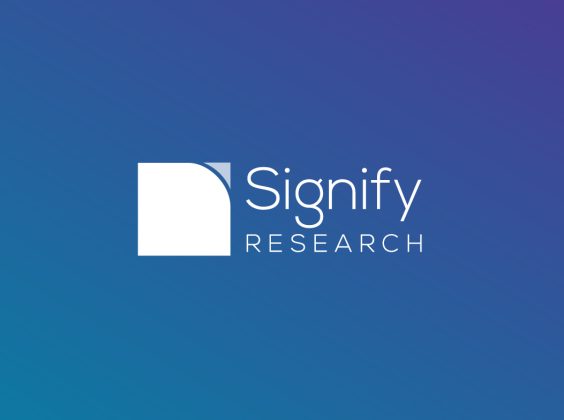
Artificial Intelligence within ultrasound
This year’s RSNA conference, as usual, was a buzzing hive of commercial activity and a feast of innovation across all imaging modalities. In particular, the energy toward Artificial Intelligence was a dominant talking point. There are clearly high expectations that AI will enable a profoundly different generation of products with the potential to unlock significant economic value in the longer-term. Within ultrasound imaging, there are some promising signs of value-adding AI solutions being brought to market. That said more advanced AI solutions, such as using AI for primary diagnosis, are still at an early stage of development and the potential opportunities to deploy this technology remain high.
Ultrasound for everybody
Ultrasound imaging is recognised as one of the fastest, safest and cheapest medical diagnostic tools available, yet it remains underused in developing markets and outside of the traditional core applications; Radiology, Cardiology and Women’s Health. As a result, there are high expectations that new users of ultrasound imaging will increase the demand for ultrasound equipment in the coming years. These new users and use-cases include primary care, first responders in emergency medical situations, anaesthetists as well as other point of care applications. For example, there is an increasing trend to carry out ultrasound-guided anaesthetic procedures, such as peripheral nerve blocks, as they can provide a more cost-effective and safer alternative to general anaesthesia. The challenge for ultrasound vendors is that many anaesthetists currently lack the specialist knowledge required to perform ultrasound-guided procedures. For ultrasound to become more accessible, vendors need to make ultrasound systems easier and more intuitive to use without compromising on scan quality. This includes providing real-time support for clinicians during scans and procedures. Some companies are using AI-technology and deep learning techniques to address this challenge through using image recognition capabilities to develop real-time training aids such as probe placement guidance and organ detection.
One of the exhibitors at RSNA, Medaphor, is developing a product which uses machine learning algorithms and deep learning techniques to automatically assess ultrasound images. Their solution is designed to guide sonographers in real-time during obstetric scans and is one of the world’s first AI-based software solutions to support sonographers in this way. The firm has acquired over one million high-quality images to train its AI algorithms, a common barrier for many developers, as well as having recently raised £7.5m to continue developing its AI software solutions for ultrasound.
Real-time guidance for probe placement will be particularly challenging due to the complexity of imaging data, individual patient considerations and inter-operator variability. The market is starting to develop solutions to address some of these more technically challenging possibilities, however they are at an early stage of development and accurate scan reads as well as scan quality remain a concern for regulators. Ultimately, the longer-term outcome is that AI could enable ultrasound to become accessible to anyone and everyone, although there are sizeable technical challenges and commercial hoops to jump through before this vision can be realised.
We envisage that in the short-term, a greater number of point solutions will be developed that provide deep expertise and a high degree of capability for specific use-cases or single applications, e.g. vessel detection. In the longer-term a more holistic artificial intelligence solution could be developed which would be capable of integrating across many ultrasound platforms as well as having the breadth and depth of medical expertise to assist with probe placement in a wider range of clinical scenarios. We envisage that it will take several years for this type of AI solution to become a reality as developing this would be a significant undertaking and require substantial financial and technical resources.
Are you seeing what I am seeing?
We are now starting to see AI and deep learning capabilities being deployed to provide anatomy-awareness where specific body parts can be automatically recognised. This image recognition capability is opening the possibility of contextually-aware systems which can assist sonographers in real-time through suggesting relevant tools as well as providing diagnostic or decision-support. For experienced sonographers, this will make relevant tools and applications more readily accessible within the workflow. For in-experienced users these real-time aids might be as simple as communicating which organ or body part is being scanned, providing support on how to best position the probe and guiding the user on the anatomical features relevant to the scan.
At RSNA, Philips Healthcare was demonstrating its Anatomical Intelligence capabilities which is designed to assess a patient’s ultrasound data and provide the sonographer with increased anatomical awareness during scans. Their ‚ÄòAI Breast’ solution enables the sonographer to identify key anatomical landmarks which helps contribute toward making more confident and reliable diagnosis. It also is capable of auto-annotation of pathologies to reduce labelling errors and deliver workflow efficiencies. We expect to see more solutions soon geared toward delivering operational efficiencies, reducing user variability and improving consistency between scans. These areas are some of the low hanging fruits as they involve easier technical challenges to solve, do not typically require FDA approval and deliver obvious economic benefits to customers.
You diagnose, whilst AI detects
The potential for utilising AI within the detection and diagnosis phases of the ultrasound imaging workflow remains high and solutions are still at a relatively early stage of development. The core drivers behind deploying AI within detection and diagnosis are focused on increasing productivity and diagnostic confidence as well as improving the ease of use for new users. Some early studies have indicated that integrating AI could improve the performance of a less-experienced radiologist to nearly the level of an expert[1], creating high future expectations for the impact of AI here.
To date, most of the solutions available to medical practitioners have been utilising AI technology to provide detection support as a second read solution. This enables radiologists to compare their findings with automated detection software powered by AI. Research is starting to show that whilst this delivers improved diagnostic accuracy, integrating AI detection concurrently with a radiologist’s appraisal of images can further improve diagnostic accuracy. This is the current frontier for AI-based detection but there is significantly more value to be unlocked here, including using AI for the primary read. Whilst there are significant potential benefits for deploying AI here, there are no such solutions for ultrasound that are commercially available today and it is likely to be several years before these solutions come to market.
As there continues to be an emerging market for the use of ultrasound in breast imaging, there is significant opportunity to utilise AI technology to address some of the main limitations of ultrasound for this application to drive increased up-take and new user sales. The main limitations of ultrasound for breast imaging include lengthy exam and reading times, relatively high number of false positives and the lack of repeatability of results due to operator dependence. Some vendors have already identified the opportunity to deploy AI-powered solutions to address these limitations and various propositions have been brought to market.
This includes QView Medical, which was showcasing its QVCAD product at RSNA. This is a CAD software system which uses AI to provide a concurrent automated detection capability during ABUS exams. Using deep learning techniques, the solution is designed to automatically detect suspicious areas of breast tissue as well as highlighting areas to distinguish potentially malignant lesions. This solution aims to reduce scan times whilst also maintaining diagnostic confidence. Samsung Medison was again showcasing its S-Detect software solution, which was first announced in 2016. S-Detect for Breast offers both detection and classification according to BI-RADS but is only FDA cleared for shape and orientation descriptors, illustrating the significant challenges of the regulatory requirements to bring these types of solutions to market. Koios was also presenting its Decision Support (DS) solution for breast ultrasound that assesses the likelihood of malignancy based on the BI-RADS evaluation scale. GE Healthcare showed Koios’ DS solution embedded on its LOGIQ E10 premium ultrasound platform as a proof of concept.
These are the pioneers and first generation of products utilising AI-technology within ultrasound detection and diagnosis, with the emphasis on detection. This remains a significant opportunity area and we expect to see greater predictive capabilities built into future solutions as well as options for a wider range of clinical applications e.g. thyroid and liver.
The ultimate step for AI within this part of the workflow will be to arrive at a credible and accurate diagnosis independent of the radiologist. This type of solution is plausible although there are significant hurdles to overcome first, including clinical validation, regulatory approval as well as successful integration across existing ultrasound platforms. There are still many unanswered technical challenges, including how well algorithms and neural networks trained using localised datasets will perform when applied to wider populations. At the same time, developers are realising that algorithms may work effectively on one OEM system, however when applied to other systems the AI fails to provide credible results. This is a real practical challenge for equipment agnostic AI software developers to overcome.
Quantification
Most major ultrasound OEMs already offer systems with embedded quantification tools for applications that require measurements, for example within cardiovascular and women’s health applications. Automating the quantification of parameters can help to deliver productivity gains through reduced assessment times, improved repeatability of assessments as well as a reduction in inter-operator variability. Reducing repetitive and time-consuming calculations to a ‘one click’ solution using non-AI based software has been on the market for some time now, although we expect to see a greater use of AI-powered quantification tools and for a wider range of clinical applications. Two examples of RSNA attendees developing AI-powered quantification include DiA Imaging Analysis and GE Healthcare. DiA provides specialist AI-powered ultrasound analysis tools, such as those used for ejection fraction calculation and analysis. GE Healthcare was showcasing SonoCNS Fetal Brain, a deep learning application providing quantification measurements of the foetal brain on its Voluson E10 ultrasound system. Over time we expect that quantification tools will be combined with detection tools, leading to quicker and more accurate diagnosis for patients.
Connecting the dots
Due to the potential breadth of specialist AI-based applications as well as the deep financial resources required to develop them, it is unlikely that one company will build everything. Instead, we expect to see more partnerships between specialist image analysis companies and ultrasound OEMs. A recent example of this is GE Healthcare’s partnership with DiA Imaging Analysis which enables users of GE’s Vscan Extend‚Ñ¢ handheld product to utilise DiA’s capabilities within cardiac decision support.
We are seeing a movement toward an ‘ecosystem’ or ‘marketplace’ approach for the distribution of AI applications into general radiology and we expect to see a similar trend for ultrasound applications. As the market evolves, we expect to see dedicated ultrasound app stores emerge as this model addresses two crucial challenges for algorithm developers – route to market and effective workflow integration. A marketplace approach would enable medical institutions to purchase and access ultrasound imaging applications tailored to their needs from one source, rather than from several vendors. Whilst we are seeing momentum toward this approach, one of the challenges and concerns about a vendor agnostic marketplace is the heightened need for cyber security in order to protect institutions from cyber-attacks and data theft. To date, the software installed on ultrasound platforms has mainly been OEM proprietary solutions, or solutions licensed from third-parties. The market has been very proprietary driven to date and for the trend toward ultrasound app marketplaces to gain traction we would need to see the OEMs open their platforms – a markedly different approach.